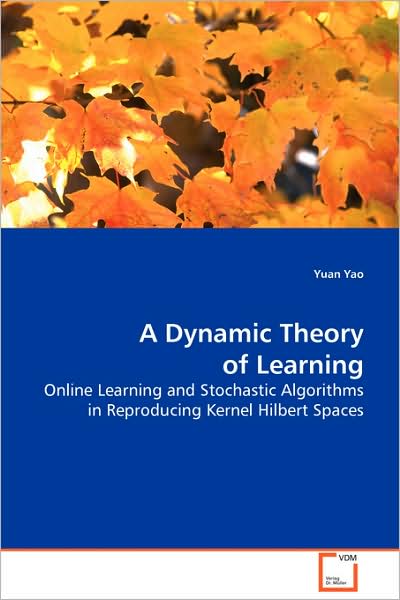
Tell your friends about this item:
A Dynamic Theory of Learning: Online Learning and Stochastic Algorithms Inreproducing Kernel Hilbert Spaces
Yuan Yao
A Dynamic Theory of Learning: Online Learning and Stochastic Algorithms Inreproducing Kernel Hilbert Spaces
Yuan Yao
In this thesis, a dynamic theory of learning, alsocalled ``online learning'' in computer science, is presented as stochastic approximations of theregression function from reproducing kernel Hilbertspaces (RKHS). It starts from a probability measureon an input-output space, with sequential sampling inan independent and identically distributed way. Online learning algorithms recursively exploitsamples as a departure from the ``batch learning''which has an access to all data once. The algorithmsare based on stochastic approximations of theregression function from RKHS. Novel probabilisticexponential inequalities in Hilbert spaces fromRussian school are exploited to study some martingaleor reverse martingale expansions of the error. Tightprobabilistic upper bounds are obtained in the sensethat in certain range of complexity classes, onlinelearning algorithms achieve the same convergencerates as batch learning, and thus asymptoticallyreach the optimal rates in some senses.
Media | Books Paperback Book (Book with soft cover and glued back) |
Released | October 29, 2008 |
ISBN13 | 9783639093902 |
Publishers | VDM Verlag |
Pages | 108 |
Dimensions | 154 g |
Language | English German |
See all of Yuan Yao ( e.g. Paperback Book )